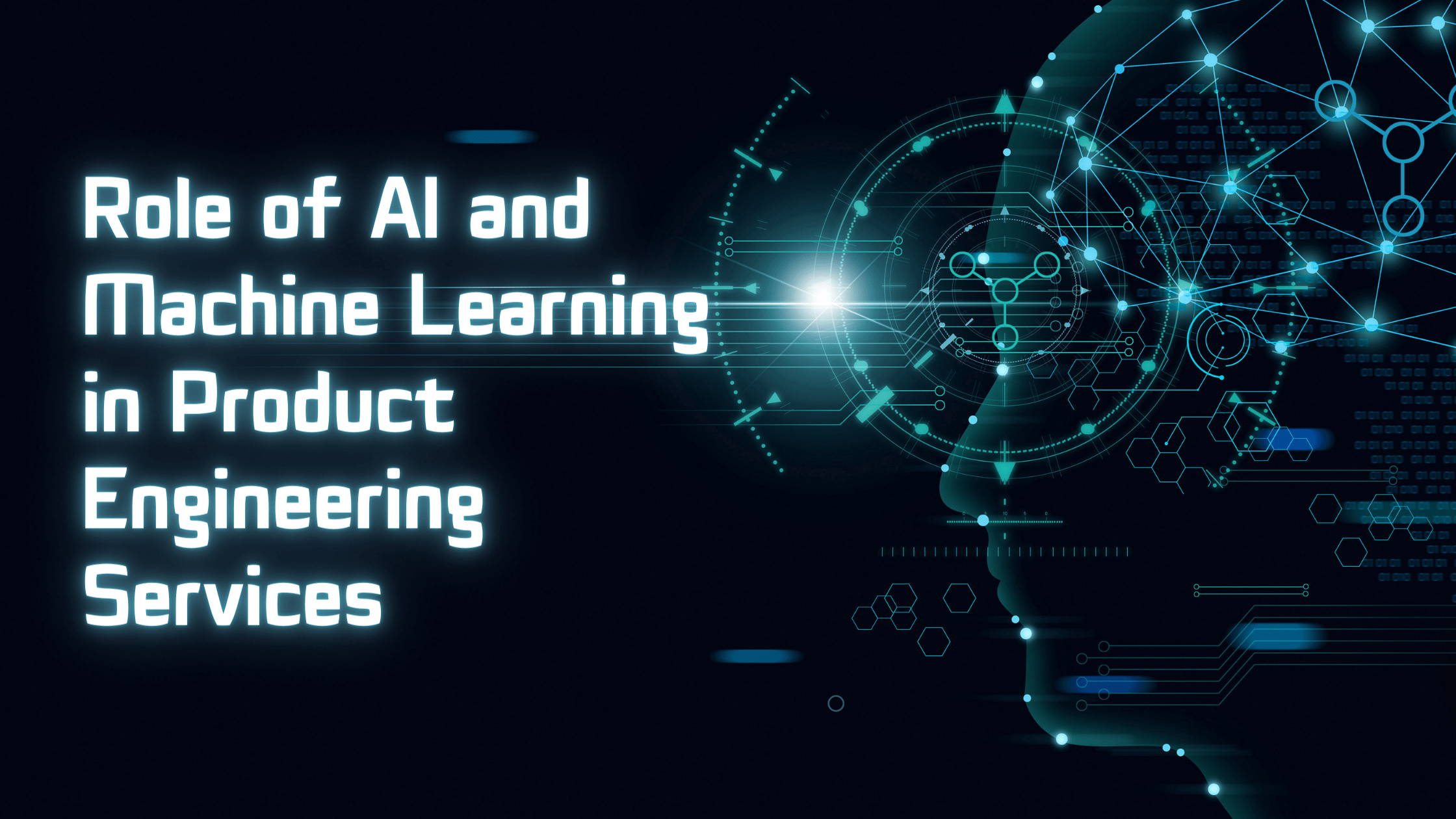
Role of AI and Machine Learning in Product Engineering Services
Introduction
In the dynamic landscape of technological advancements, Artificial Intelligence (AI) and Machine Learning (ML) have become synonymous with innovation, reshaping industries globally. Their profound impact on product engineering services is a testament to their transformative capabilities. This article explores the evolution, current landscape, benefits, applications, challenges, future trends, and case studies of AI and ML in product engineering, offering insights into their pivotal role in shaping the future of innovation.
Artificial Intelligence (AI) and Machine Learning (ML) have emerged as game-changers in the world of technology. AI involves the simulation of human intelligence in machines, while ML, a subset of AI, empowers systems to learn from data. The integration of these cutting-edge technologies into product engineering services in the USA has ushered in a new era of possibilities and efficiency.
Overview of Product Engineering Services
Product engineering services encompass the entire product development lifecycle, from conception to deployment. The intricacies involved in this process demand innovative solutions, and AI and ML have proven to be indispensable tools in overcoming these challenges. They contribute to accelerating development cycles, improving product quality, and optimizing overall performance.
Evolution of AI and Machine Learning in Product Engineering
The historical development of Artificial Intelligence (AI) and Machine Learning (ML) within the realm of product engineering marks a captivating journey that spans decades. In the mid-20th century, the inception of AI was more theoretical than practical, with visionaries envisioning machines emulating human intelligence. The 1970s witnessed a pivotal milestone with the advent of expert systems, laying the groundwork for intelligent decision-making in product engineering.
The subsequent years saw AI navigating through peaks and troughs until the 21st century, where the resurgence of neural networks brought about a revolutionary shift. ML, a subset of AI, gained prominence as algorithms evolved to learn patterns and insights from data, a capability with profound implications for product engineering.
Key milestones include the development of expert systems, symbolic reasoning, and the breakthroughs in neural network research. These milestones collectively paved the way for the seamless integration of AI and ML into the intricate fabric of product engineering, transforming the industry’s approach to innovation and problem-solving.
Current Landscape
In the USA and worldwide, product engineering services companies are seamlessly integrating AI and ML into various facets of the process. From automating routine tasks to facilitating complex decision-making processes, these technologies play a pivotal role. For instance, ML algorithms are utilized in supply chain optimization, ensuring the timely and cost-effective delivery of materials. This integration exemplifies the practical application of AI and ML in real-world scenarios.
Benefits of AI and Machine Learning
The advantages of incorporating AI and ML into product engineering are multifaceted. One of the primary benefits is the enhancement of efficiency across the entire development lifecycle. By automating repetitive tasks, these technologies allow human resources to focus on more strategic and creative aspects of the engineering process. Improved quality control is another significant advantage, with ML algorithms identifying defects with unparalleled precision. Additionally, many product engineering services companies in the USA aim at substantial cost savings that are achieved through the optimization of processes and resources.
Applications in Product Engineering
In the dynamic landscape of product engineering, the integration of Artificial Intelligence (AI) and Machine Learning (ML) has led to transformative applications, redefining traditional processes. Three standout use cases—predictive maintenance, design optimization, and quality control—exemplify the profound impact of these technologies.
Predictive Maintenance: AI and ML algorithms analyze historical and real-time data to forecast equipment failures. This proactive approach reduces downtime and optimizes operational efficiency. A manufacturing plant’s implementation of predictive maintenance resulted in a 30% reduction in maintenance costs and a notable 20% increase in equipment uptime.
Design Optimization: AI and ML enable engineers to leverage data-driven insights for refining product designs. Algorithms analyze vast datasets to generate optimized designs, prioritizing factors like performance and cost-effectiveness. A consumer electronics company’s use of ML for design optimization led to a remarkable 15% improvement in overall product performance.
Quality Control: AI and ML-driven quality control processes enhance precision in defect identification. Automation ensures products meet stringent standards, reducing the likelihood of defects reaching end-users. A manufacturing facility’s implementation of ML-driven quality control resulted in decreased defective products and an overall improvement in product quality.
These applications showcase the tangible benefits of AI and ML in enhancing efficiency, reducing costs, and elevating product quality in the field of product engineering. As technology advances, these applications are poised to further revolutionize the industry.
Challenges and Considerations
While the transformative potential of AI and ML is undeniable, challenges and ethical considerations must be addressed. One prominent challenge is the presence of bias in algorithms, which can perpetuate and even exacerbate existing inequalities. Data privacy concerns also loom large, as the increasing reliance on data for training AI models raises questions about the security and confidentiality of sensitive information. Job displacement is another consideration, as automation may lead to shifts in workforce dynamics.
Mitigating these risks involves proactive measures such as establishing ethical guidelines for AI development and deployment. Transparent algorithms and practices can help address bias concerns, ensuring that AI systems are fair and inclusive. Workforce upskilling and reskilling programs can also play a crucial role in preparing employees for the evolving nature of their roles in a technology-driven landscape.
Future Trends
Looking ahead, natural language processing and computer vision are poised to revolutionize AI and ML applications in product engineering. These technologies will enable machines to understand and interpret human language and visual information, opening new frontiers in product development and user interaction. The ability to analyze and respond to natural language queries and interpret visual data will further enhance the user experience and streamline product development processes.
Conclusion
In conclusion, the amalgamation of AI and ML with product engineering services in the USA and across the world represents a paradigm shift in innovation. The historical evolution, current applications, and future trends highlight the transformative potential of these technologies. As the landscape continues to evolve, addressing challenges and ethical considerations becomes imperative.
The benefits of enhanced efficiency, improved quality, and substantial cost savings underscore the inevitability of AI and ML becoming indispensable tools in the product engineer’s toolkit. Embracing these technologies is not merely a choice; it is a necessity for those seeking to lead the way in the ever-evolving landscape of product engineering. The journey towards innovation and excellence in product engineering services companies are now intricately linked with the integration of latest technology, promising a future where efficiency, quality, and creativity converge to shape groundbreaking products.