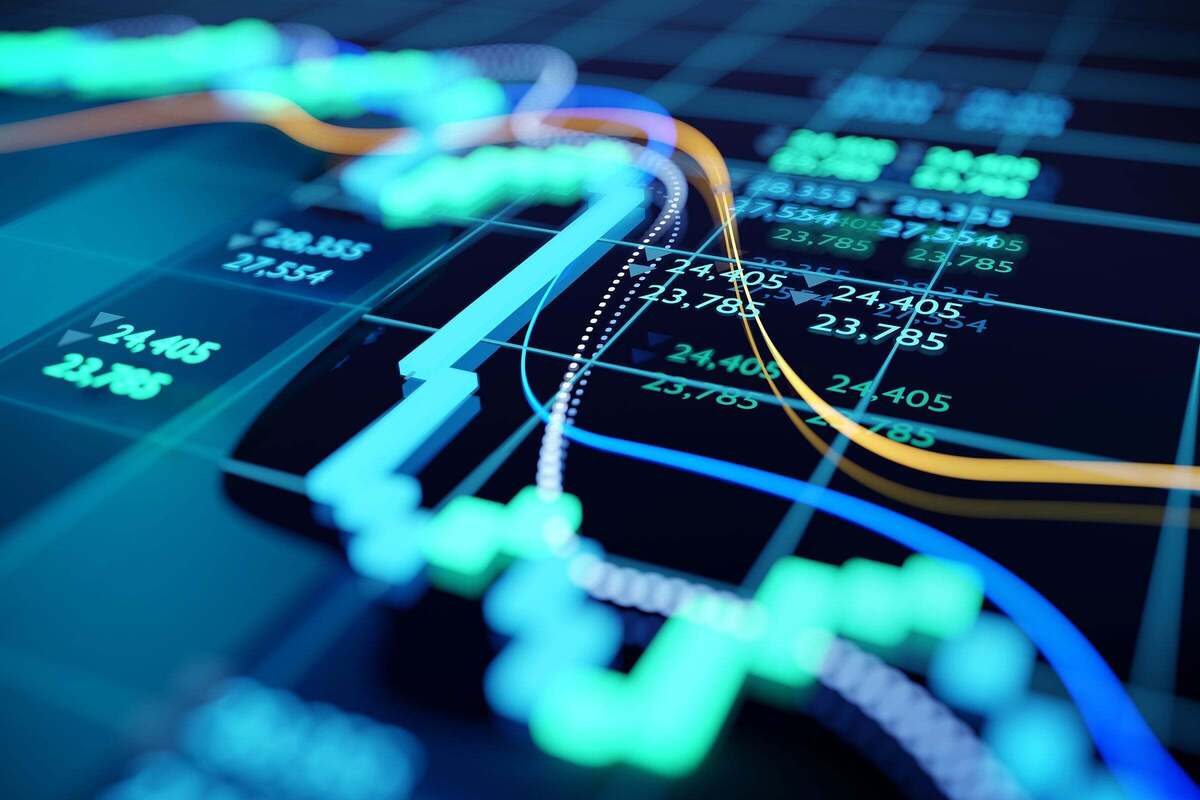
Predictive networking claims a lot quicker fixes
[ad_1]
With the support of artificial intelligence (AI) and device learning (ML), predictive network engineering alerts directors to possible network difficulties as early as achievable and features opportunity solutions.
The AI and ML algorithms utilized in predictive network know-how have become essential, suggests Bob Hersch, a principal with Deloitte Consulting and US lead for platforms and infrastructure. “Predictive community technological innovation leverages synthetic neural networks and makes use of designs to analyze information, master designs, and make predictions,” he says. “AI and ML drastically enhance observability, application visibility, and the skill to reply to community and other problems.”
Though predictive community technological know-how has produced spectacular strides above the earlier many yrs, several developers and observers are self-confident that the most effective is nonetheless to come. “Applications and devices are out there now, but like most important evolutions in know-how there are risks for the early adopters, as enhancement and even how to assess the performance of a change are in flight,” states David Lessin, a director at know-how investigation and advisory agency ISG.
Predictive analytics is no more time just for predicting community outages and proactively managing difficulties of bandwidth and application functionality, claims Yaakov Shapiro, CTO at telecommunications program and products and services provider Tangoe. “Predictive analytics are now currently being utilized to complications bordering the network and helping to tackle the downsides of SD-WAN, most notably the problem of service provider sprawl and the want for wider provider-services administration and telecom-price optimization,” he states. “These have turn into larger challenges in the age of buying and selling MPLS—one- and two-provider services—for broadband solutions comprising likely hundreds of web provider providers.”
AI is going predictive networking forward.
The most latest evolution of AI is the most important advancement in predictive community technology. “Cloud-centered AI systems can increase the excellent and velocity of data delivered to community technicians even though supplying them a valuable device to look into outages and other challenges,” states Patrick MeLampy, a Juniper Networks fellow. “AI can detect anomalies a lot quicker than human beings and can even review the root induce of an anomaly, serving to to guide a technician to comprehend and restore the difficulty quicker than ahead of.”
The integration of AI applications into predictive community engineering also has the possible to be an financial video game-changer. “With experienced AI and ML equipment at their disposal, service suppliers and corporations alike can lessen the expenditures of problem discovery and resolution,” MeLampy suggests. In addition to bottom-line financial rewards, AI can help to simplify management, either inside an business or throughout a provider provider’s portfolio. “Mean-time-to fix is reduced, improving conclude consumer fulfillment as very well,” he suggests.
Bryan Woodworth, principal answers strategist at multicloud community technological know-how business Aviatrix, claims that predictive community technological know-how will progress rapidly around the next couple of yrs. It presently aids solve community problems immediately and effectively. “AI can correlate alerts and mistake disorders across a lot of disparate methods, getting linked styles in minutes or even seconds, something that would take individuals several hours or days,” he claims.
Predictive community technologies can also considerably lower the selection of wrong positives tucked into log and mistake analyses, leading to a lot more clever and helpful alerts, Woodworth states. “You won’t be able to heal from anything you do not detect,” he claims. “For case in point, prior to you change the network to route all around a problem, you must know where by that problem is.” Self-therapeutic networks centered on AI and ML deliver much better tips on how to get well from mistakes and steer clear of outages.
Predictive modeling is effective finest in info centers.
Network conduct analytics examines community info, such as ports, protocols, performance, and geo-IP facts, to warn whenever you can find been a significant alter in community actions that may suggest a threat. “In the foreseeable future, this knowledge can be fed into an AI product that can support verify if the danger is authentic, and then make strategies on how to remediate the concern by transforming the network,” Woodworth states. “This form of predictive modeling will work greatest inside private networks, like the knowledge heart, simply because [that’s where] individuals have complete management above all the networking components and the details they make.”
For public networks, together with those people connected to the net, the job turns into far more difficult. Learning styles have to be made to compensate for systems that aren’t below direct command or offer incomplete information sets. This suggests that learning types will make less accurate predictions and may need to be tuned by humans to compensate for the missing facts, Woodworth claims.
To be completely helpful, sophisticated AI and ML models should really operate at manufacturing amount and scale for error remediation, Smith suggests. “Determination-makers will need to rely on modeling effects, and engineering sponsors will need to execute functions effectively,” he states.
Meanwhile, ongoing advances in cloud technological know-how and graphics processing units (GPUs) are taking modeling to new degrees. “Open up source and professional frameworks are supporting corporations deploy ML operations quickly and at-scale with a lot less threat related with the time and complexity demanded to configure cloud and open up supply techniques for AI,” claims Maggie Smith, controlling director, used intelligence, at consulting agency Accenture Federal Companies.
Smith suggests that quite a few key cloud suppliers have already executed AI design optimization and management features. The technological know-how can be observed in in applications this kind of as Amazon SageMaker, Google AI Platform, and Azure Device Mastering Studio. “Open-resource frameworks like TensorRT, and Hugging Deal with retrain more chances for product checking and efficiencies,” Smith claims.
Predictive networking analyzes cloud and edge workloads.
Large image, predictive AI-based networking is not as much about the network as it is about cloud workloads, edge supply, and person endpoint products, these types of as laptop computer computer systems and cell gadgets. “By knowing workloads—the network targeted traffic they crank out, latency requirements, and who is consuming facts how and where—the superior-fidelity details necessary for predictive networking can be identified to help the automated adaptation of virtual private clouds (VPCs),” states Curt Aubley, threat and financial advisory handling director, and US cyber detect-and-respond leader at company advisory company Deloitte.
Micro segmentation, load balancers, and targeted visitors shapers are all serving to to optimize supply. “The same superior-fidelity information made use of for network-concentrated AI can also be used to enhance cyber-security teams’ consolidated prolonged detection and response data lakes for stability analytics,” Aubley states. AI models are used to detect anomalies, unidentified unknowns, and lateral movement. “Working with the identical higher-fidelity details from cloud workloads, networks, and endpoints for distinctive use situations can enable make sure confidentiality, integrity, and the availability of purposes required for enterprise or federal government cyber possibility management.”
Routers, wi-fi applications, switches, and different other general networking equipment really don’t typically collect person-particular info. Though software-functionality monitoring instruments do measure consumer info, they are unable to correlate benefits into proactive network steps. “Networks should turn out to be person and application informed in get to collect the types of information necessary to create actionable versions for the use of AI and predictive technologies,” MeLampy suggests. “If a alternative will not measure working experience for each consumer, it is just not heading to be effective.”
Prescriptive analytics is the long run.
The emerging field of neuromorphic computing, centered on a chip architecture that is engineered to mimic human mind framework, promises to provide remarkably productive ML on edge products. “Predictive network technology is so effective since of its potential to intake indicators and make exact predictions about devices failures to optimize routine maintenance,” suggests Gil Dror, CTO at checking technological innovation provider SmartSense. He claims that neuromorphic computing will develop into even much more strong when it moves from predictive to prescriptive analytics, which endorses what should be finished to assure upcoming results.
Neuromorphic computing’s chip architecture is geared towards creating clever choices on edge gadgets themselves, Dror states. “The mix of these two technologies will make the discipline of predictive network engineering a lot a lot more potent,” he claims.
Organizations including IBM, Intel, and Qualcomm are building neuromorphic computing technologies. “Some corporations have produced neuromorphic computing chips for investigation-and-growth functions, this kind of as IBM’s TrueNorth chip and Intel’s Loihi chip,” Dror says. These chips usually are not yet generally offered for industrial use, and it can be most likely that there will be at the very least quite a few much more decades of rigorous research and enhancement before neuromorphic computing becomes a mainstream engineering. “When it will become practical, the impact will be substantial,” he predicts.
Copyright © 2023 IDG Communications, Inc.
[ad_2]
Source hyperlink