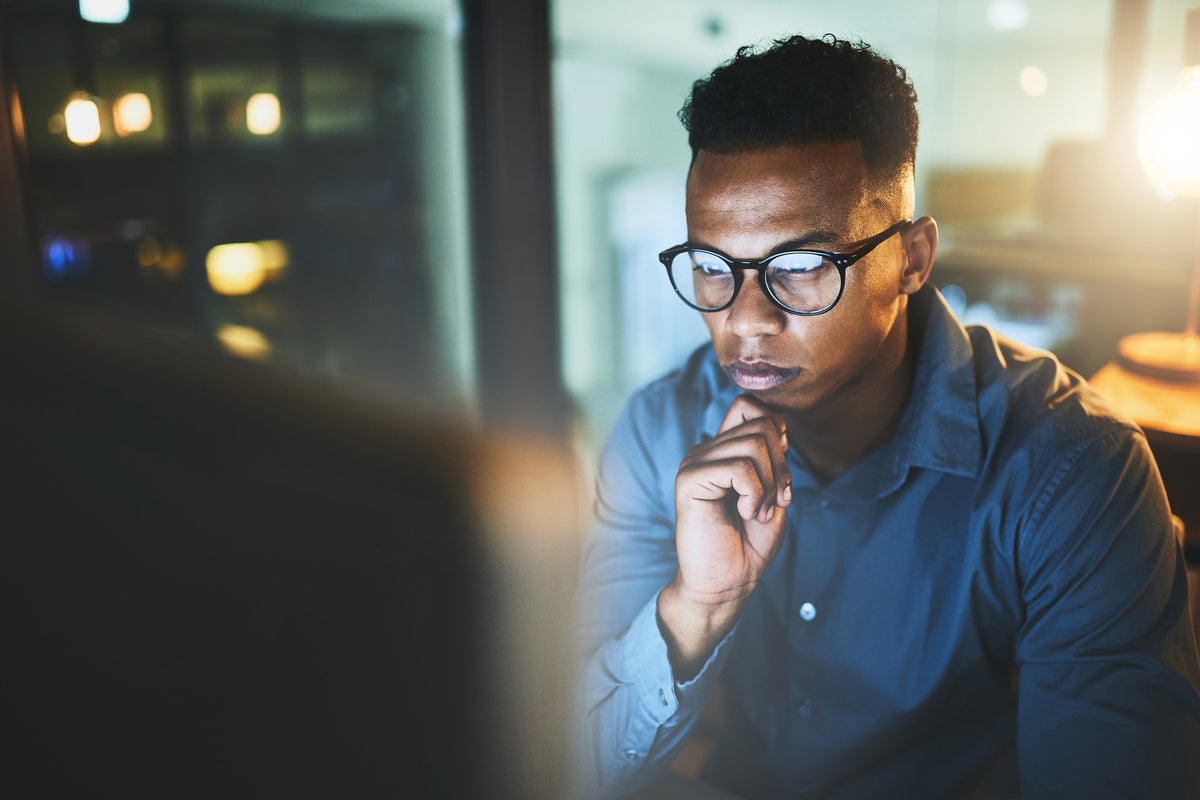
How IT professionals may discover to imagine in AI-driven community-management
[ad_1]
IT companies that apply artificial intelligence and machine understanding (AI/ML) technology to community management are getting that AI/ML can make faults, but most businesses believe that that AI-driven community management will strengthen their network operations.
To know these gains, community professionals need to come across a way to have faith in these AI alternatives even with their foibles. Explainable AI applications could hold the key.
A survey finds network engineers are skeptical.
In an Business Management Associates (EMA) study of 250 IT pros who use AI/ML know-how for network management, 96% stated all those remedies have manufactured false or mistaken insights and suggestions. Almost 65% described these problems as relatively to really unusual, according to the latest EMA report “AI-Driven Networks: Leveling Up Community Management.” General, 44% % of respondents mentioned they have sturdy have confidence in in their AI-driven network-management applications, and a further 42% a little bit trust these equipment.
But users of community-engineering teams described more skepticism than other groups—IT device engineers, cloud engineers, or customers of CIO suites—suggesting that folks with the deepest networking expertise were being the least convinced. In point, 20% of respondents claimed that cultural resistance and distrust from the network crew was one of the most important roadblocks to prosperous use of AI-pushed networking. Respondents who operate inside a network engineering workforce were two times as most likely (40%) to cite this problem.
Given the prevalence of errors and the lukewarm acceptance from large-level networking professionals, how are businesses developing have confidence in in these options?
What is explainable AI, and how can it aid?
Explainable AI is an tutorial strategy embraced by a increasing quantity of suppliers of professional AI alternatives. It’s a subdiscipline of AI investigate that emphasizes the development of tools that spell out how AI/ML technology helps make decisions and discovers insights. Scientists argue that explainable AI tools pave the way for human acceptance of AI know-how. It can also address issues about ethics and compliance.
EMA’s exploration validated this notion. More than 50% of study members stated explainable AI instruments are really crucial to making have confidence in in AI/ML engineering they implement to network administration. An additional 41% explained it was rather essential.
Majorities of contributors pointed to three explainable AI equipment and techniques that ideal assist with creating have confidence in:
- Visualizations of how insights were being found out (72%): Some suppliers embed visual elements that information people as a result of the paths AI/ML algorithms consider to create insights. These contain selections trees, branching visible aspects that show how the technologies is effective with and interprets network information.
- Purely natural language explanations (66%): These explanations can be static phrases pinned to outputs from an AI/ML instrument and can also come in the type of a chatbot or virtual assistant that presents a conversational interface. Consumers with varying stages of technological know-how can recognize these explanations.
- Chance scores (57%): Some AI/ML solutions current insights without context about how confident they are in their have conclusions. A probability score takes a distinct tack, pairing each perception or recommendation with a score that tells how self-confident the system is in its output. This assists the person ascertain no matter whether to act on the data, get a hold out-and-see strategy, or dismiss it entirely.
Respondents who noted the most overall accomplishment with AI-driven networking options were being far more probable to see value in all a few of these abilities.
There may be other approaches to develop believe in in AI-pushed networking, but explainable AI could be a person of the most effective and productive. It gives some transparency into the AI/ML units that could or else be opaque. When evaluating AI-pushed networking, IT consumers must check with sellers about how they support operators develop trust in these programs with explainable AI.
Copyright © 2023 IDG Communications, Inc.
[ad_2]
Source hyperlink