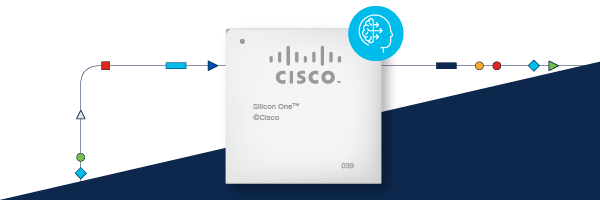
Building AI/ML Networks with Cisco Silicon One
[ad_1]
It’s apparent from the amount of information protection, posts, blogs, and h2o cooler tales that synthetic intelligence (AI) and equipment finding out (ML) are modifying our culture in basic ways—and that the marketplace is evolving immediately to try to retain up with the explosive development.
Regretably, the community that we’ve utilized in the earlier for high-effectiveness computing (HPC) are unable to scale to meet up with the calls for of AI/ML. As an field, we must evolve our imagining and build a scalable and sustainable network for AI/ML.
Now, the sector is fragmented in between AI/ML networks built all-around four exclusive architectures: InfiniBand, Ethernet, telemetry assisted Ethernet, and absolutely scheduled materials.
Each and every engineering has its pros and disadvantages, and several tier 1 web scalers view the trade-offs otherwise. This is why we see the industry shifting in a lot of instructions simultaneously to satisfy the swift massive-scale buildouts happening now.
This reality is at the coronary heart of the benefit proposition of Cisco Silicon A single.
Consumers can deploy Cisco Silicon A single to electric power their AI/ML networks and configure the community to use regular Ethernet, telemetry assisted Ethernet, or completely scheduled fabrics. As workloads evolve, they can continue to evolve their contemplating with Cisco Silicon One’s programmable architecture.

All other silicon architectures on the market place lock organizations into a narrow deployment model, forcing buyers to make early purchasing time choices and limiting their overall flexibility to evolve. Cisco Silicon A single, on the other hand, offers buyers the overall flexibility to method their network into a variety of operational modes and presents most effective-of-breed characteristics in every single manner. Simply because Cisco Silicon One can permit a number of architectures, shoppers can aim on the truth of the info and then make information-pushed conclusions in accordance to their very own criteria.

To support understand the relative merits of each of these systems, it’s crucial to realize the fundamentals of AI/ML. Like many buzzwords, AI/ML is an oversimplification of many exclusive systems, use instances, targeted visitors styles, and specifications. To simplify the discussion, we’ll emphasis on two areas: coaching clusters and inference clusters.
Schooling clusters are developed to build a model utilizing acknowledged knowledge. These clusters coach the model. This is an very sophisticated iterative algorithm that is run throughout a enormous variety of GPUs and can operate for numerous months to create a new model.
Inference clusters, in the meantime, choose a educated product to evaluate unfamiliar info and infer the reply. Merely put, these clusters infer what the not known knowledge is with an presently experienced design. Inference clusters are significantly smaller computational styles. When we interact with OpenAI’s ChatGPT, or Google Bard, we are interacting with the inference versions. These styles are a end result of a very considerable education of the model with billions or even trillions of parameters over a long period of time of time.
In this blog site, we’ll concentration on schooling clusters and examine how the general performance of Ethernet, telemetry assisted Ethernet, and completely scheduled materials behave. I shared more facts about this matter in my OCP World-wide Summit, October 2022 presentation.
AI/ML teaching networks are crafted as self-contained, huge back-stop networks and have significantly different site visitors designs than traditional entrance-stop networks. These back-conclude networks are used to have specialised targeted visitors amongst specialised endpoints. In the past, they have been applied for storage interconnect, on the other hand, with the arrival of remote immediate memory access (RDMA) and RDMA more than Converged Ethernet (RoCE), a important portion of storage networks are now built in excess of generic Ethernet.
Currently, these again-end networks are currently being utilised for HPC and massive AI/ML education clusters. As we saw with storage, we are witnessing a migration absent from legacy protocols.
The AI/ML coaching clusters have distinctive targeted traffic styles when compared to conventional entrance-conclude networks. The GPUs can thoroughly saturate superior-bandwidth inbound links as they ship the effects of their computations to their friends in a knowledge transfer identified as the all-to-all collective. At the end of this transfer, a barrier procedure makes certain that all GPUs are up to date. This creates a synchronization occasion in the community that triggers GPUs to be idled, waiting for the slowest route through the network to entire. The task completion time (JCT) actions the general performance of the community to be certain all paths are doing properly.

This visitors is non-blocking and results in synchronous, significant-bandwidth, long-lived flows. It is vastly distinct from the information patterns in the front-end network, which are principally developed out of several asynchronous, small-bandwidth, and small-lived flows, with some more substantial asynchronous prolonged-lived flows for storage. These discrepancies together with the importance of the JCT signify network functionality is vital.
To analyze how these networks execute, we produced a product of a smaller education cluster with 256 GPUs, 8 major of rack (TOR) switches, and four backbone switches. We then utilized an all-to-all collective to transfer a 64 MB collective sizing and change the quantity of simultaneous positions working on the network, as very well as the volume of community in the speedup.
The benefits of the study are remarkable.
Not like HPC, which was designed for a solitary career, large AI/ML instruction clusters are intended to run numerous simultaneous careers, likewise to what takes place in net scale information facilities these days. As the selection of careers increases, the results of the load balancing plan utilised in the community grow to be far more clear. With 16 careers jogging throughout the 256 GPUs, a absolutely scheduled material benefits in a 1.9x quicker JCT.

Learning the details a further way, if we keep an eye on the total of precedence movement manage (PFC) sent from the community to the GPU, we see that 5% of the GPUs sluggish down the remaining 95% of the GPUs. In comparison, a entirely scheduled cloth provides completely non-blocking effectiveness, and the community under no circumstances pauses the GPU.

This suggests that for the similar network, you can link twice as lots of GPUs for the exact same sizing network with thoroughly scheduled fabric. The aim of telemetry assisted Ethernet is to boost the functionality of common Ethernet by signaling congestion and improving load balancing decisions.
As I outlined previously, the relative merits of numerous systems fluctuate by just about every buyer and are likely not frequent above time. I consider Ethernet, or telemetry assisted Ethernet, even though decreased effectiveness than thoroughly scheduled materials, are an very worthwhile know-how and will be deployed extensively in AI/ML networks.
So why would prospects decide on 1 know-how in excess of the other?
Clients who want to delight in the major financial commitment, open up requirements, and favorable price-bandwidth dynamics of Ethernet ought to deploy Ethernet for AI/ML networks. They can strengthen the overall performance by investing in telemetry and reducing network load by way of watchful placement of AI work on the infrastructure.
Shoppers who want to appreciate the whole non-blocking overall performance of an ingress digital output queue (VOQ), totally scheduled, spray and re-purchase material, ensuing in an amazing 1.9x far better position completion time, must deploy fully scheduled fabrics for AI/ML networks. Completely scheduled materials are also terrific for consumers who want to help save expense and electrical power by removing network aspects, however continue to achieve the same performance as Ethernet, with 2x extra compute for the very same community.
Cisco Silicon A person is uniquely positioned to give a alternative for either of these shoppers with a converged architecture and marketplace-foremost efficiency.

Master far more:
Read through: AI/ML white paper
Pay a visit to: Cisco Silicon 1
Share:
[ad_2]
Source backlink